The Main Points Of Labeling Individual Elements For Training Data In ML
by Abdul Aziz Mondal Business Intelligence 20 February 2023
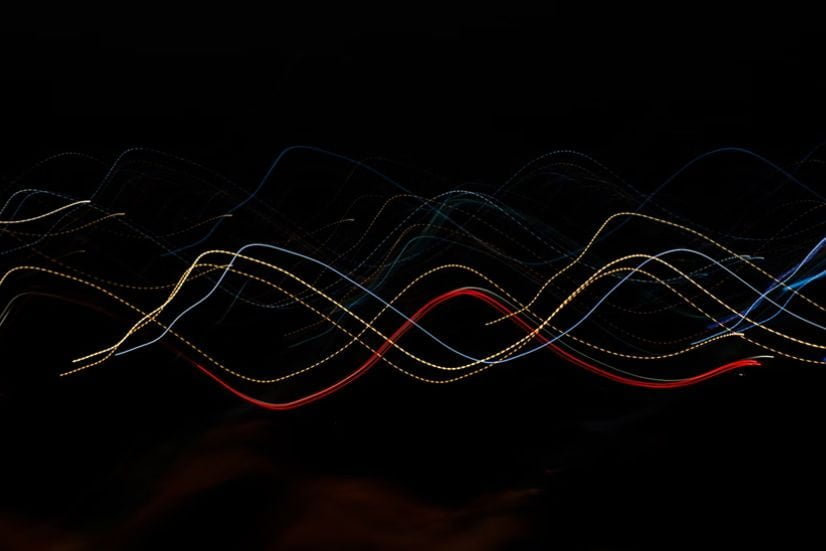
In today’s fast-paced digital world, companies are constantly seeking ways to improve their data-driven processes. Data annotation plays a significant role in achieving this goal. In essence, data labeling is the process of adding meaningful information to raw data, making it ready to be used for machine learning models. Since data labeling is a crucial aspect of developing such models, it’s important to understand the key points in this process.
The demand for data annotation services is rapidly increasing, with the market expected to reach $3 billion by 2025. With this growth comes the need for accurate and high-quality data annotations. When data is properly labeled, machine learning algorithms can be trained to identify objects and make predictions with higher precision.
In this article, we’ll explore the main factors of quality data labeling and provide best practices to ensure it. If you’re interested in improving your data-driven processes, this article is a must-read.
Mastering The Process: Why Do We Need To Ensure Data Annotation Accuracy In ML?
Inaccurately labeled data can lead to its misinterpretation, resulting in wrong predictions or decisions made by the ML model. This can have significant consequences for the most common applications of ML in real life. Imagine inserting imprecise data when dealing with disease detection on MRI scans, or mapping a road for self-driving vehicles. To avoid frightful outcomes, annotated data should be constantly checked.
Here are a few reasons that highlight the importance of correctly annotated data:
- Model performance: The quality of the labeled data directly impacts the performance of the ML model. If the data is inaccurately labeled, the model will not be able to learn meaningful patterns, resulting in poor capacity.
- Training time: Data labeling of bad quality can significantly increase the time and resources required to train the model. This is because a model trained on wrongly labeled data needs to be retrained, leading to delays and additional costs.
- Model reliability: The accuracy of the data labeling directly affects the trustworthiness of the ML model. If the data is labeled imprecisely, the model may make incorrect predictions, leading to misleading results.
- Business outcomes: Inadequate data labeling can have negative impacts on the outcomes of AI initiatives. For example, a driverless model trained on poorly labeled data may result in unsafe driving conditions and accidents.
A professional approach to data labeling, combined with the right tools and techniques, can ensure prosperous results for your ML projects. Hiring a professional data annotation company (e.g., labelyourdata.com) is often the best approach for organizations looking to be the best in their field.
Third-party companies also provide expertise and experience, scalability, and quality control, ensuring that the data labeling process is handled fast and efficiently. This results in a high-quality labeled dataset that can be used to develop an effective and trustworthy ML model.
From Elements To Outcomes: Important Factors For Perfect Data Annotation
In the data annotation process, there’s no one-size-fits-all approach. Depending on your company’s demands, you may select the strategy that meets those objectives the best way.
There are several known practices, each with its own advantages and disadvantages.
- Crowdsourcing: Crowdsourcing involves outsourcing the labeling process to a large group of individuals This method is cost-effective and allows for a quick turnaround time, but it may lead to inconsistencies in the labeling process and a lower level of quality control.
- In-house annotation: In-house annotation involves hiring a team of nota It offers more control over the quality of the labeling process and allows for more consistency, but it is often more expensive and may take longer.
- Automated annotation: Automated annotation uses machine learning algorithms to label data. It’s quick and cost-effective, but also limited by the accuracy of the algorithms and may not produce high-quality annotations.
- Hybrid approach: The hybrid approach combines elements of crowdsourcing and in-house annotation. This approach offers the cost-effectiveness of crowdsourcing while maintaining a higher level of quality control through in-house annotators.
Choosing the right data labeling workflow depends on a variety of factors, including the type and volume of data, your budget, and your desired level of quality control. Despite the approach you choose, we advise taking into account these tried-and-true aspects to manage a project successfully:
- Defined guidelines: Developing clear and concise guidelines for data annotation is the first step in ensuring remarkable outcomes. This helps ensure consistency in the annotation process, as all annotators will have a clear understanding of what is expected of them. The plan should cover things like the type of data to be annotated, the type of annotations required, and the standards to be followed.
- Qualified annotators: Choosing the right annotators for the job is another critical factor. It’s essential to have annotators who are well-trained and experienced in the domain of the data to be annotated. This ensures that the annotations are accurate and of high quality.
- Quality control: Implementing a quality control check is a key step in ensuring the completeness of the annotation. This can be done by having multiple annotators annotate the same data, or by using automated quality control tools. The goal of quality control is to identify any inaccuracies in the annotations and correct them before the data is used for training the algorithms.
- Quality assurance: There are numerous methods that can be used to find and correct errors in data annotation. These methods guarantee that the data that is actually delivered is of the best standard, accuracy, and reliability.
- Regular review: Regularly reviewing the data annotation process is another important factor. This allows you to identify any issues with the workflow and make improvements to ensure clarity. The review should include checks on the compliance and accuracy of the annotations, as well as identifying any issues with the guidelines or the annotators.
- Consistent annotation process: Maintaining uniformity in the annotation process is crucial. Inconsistent annotations can result in the failure of the whole project. To ensure consistency, it’s fundamental to have a well-defined set of rules that is followed by all annotators.
Final Thoughts
The rapid growth of AI is driving the need for more advanced technologies and systems to simplify our lives. Data labeling remains the main way for obtaining precise training datasets for ML models. However, as the volume of data used for algorithms increases, it becomes challenging to ensure that the labeled data is of the highest quality.
Reduced model performance and unreliable results are just some of the consequences of poor data labeling, which can be hazardous. But there are proven ways to avoid them. From hiring experienced annotators to implementing quality control methods, here we’ve covered the key steps to master the labeling workflow and achieve successful outcomes for your AI initiatives.
Read Also: