Data Mining Services Dilemmas: Tackling Complex Problems And Roadblocks
by Debamalya Mukherjee Technology Published on: 28 November 2023 Last Updated on: 06 December 2023
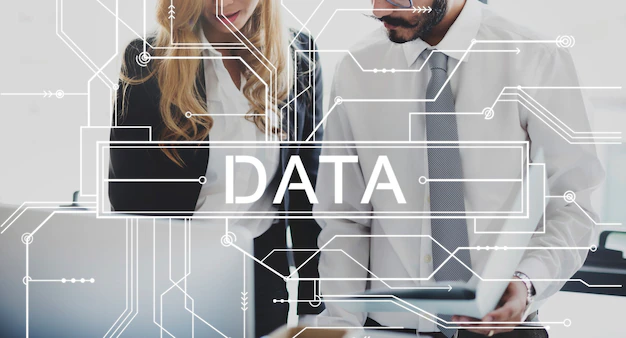
From determining whether the new product/idea will work in the market to deciding what type of ad campaign would have a considerable impact on the audience—data-driven insights are what keep businesses up and running today, fueling majority decisions, processes, and actions.
More like a knowledge discovery process, data mining has become an indispensable tool for organizations across various industries including eCommerce, retail, healthcare, manufacturing, banking, finance, and so on. Having said that, investing in data mining services can be immensely beneficial for companies looking to extract valuable insights from large datasets.
Navigating the Challenges in Data Mining
Despite its immense potential, data mining comes with its set of dilemmas and challenges that organizations must carefully navigate to ensure ethical and effective data utilization.
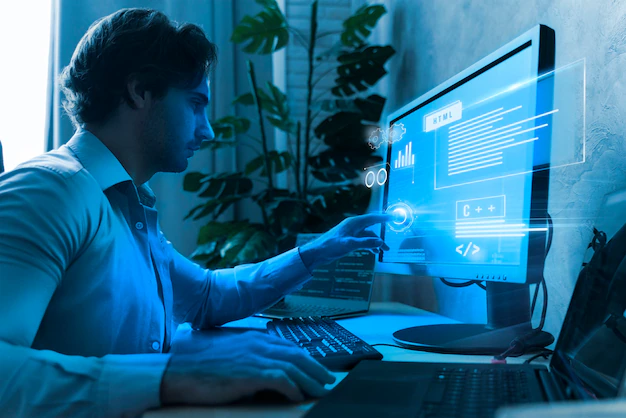
Let’s take a look at some of the major roadblocks in the data mining process:
1. Data Quality
The quality of data being mined plays a significant role in ensuring the accuracy and reliability of the outcomes. Poor-quality or erroneous data can lead to inaccurate or unreliable insights. Moreover, the data might be incomplete, meaning that some values or attributes might be missing, making it difficult to obtain a complete understanding of it. Thus, it is important to keep a check on the completeness, coherence, and consistency of the data used for mining.
Data quality problems may emerge due to a variety of reasons such as errors in data entry, issues with data storage, problems in data integration, and errors in data transmission. To overcome these challenges, data mining service providers must implement data quality assessment and cleansing processes. Data profiling tools can be used to identify anomalies and inconsistencies whereas regular audits and cleansing can help in enhancing its quality.
2. Data Integrity
Along with data comes the challenge of maintaining its security and integrity. As more data is pooled, processed, analyzed, and stored, the risk of cyber-attacks and data breaches increases. Handling sensitive or personal information raises privacy issues and may lead to ethical and legal challenges. Privacy regulations such as CCPA, GDPR, HIPAA, etc. impose restrictions on how data can be collected, used, or shared.
Prioritizing data anonymization and encryption can help safeguard the integrity of the data. Data anonymization involves removing Personally Identifiable Information (PII) from the data, whereas encryption involves using Machine Learning algorithms to encode the information to make it unreadable to unauthorized users.
3. Data Complexity and Integration
True data is heterogeneous in nature as it is derived from various sources, including social media, sensors, and the Internet of Things (IoT). It is generated in multiple formats such as natural language text, spatial data, time series, temporal data, audio, or video, images, etc., making it difficult to integrate a single dataset. Processing, analyzing, and understanding such complex data without missing its essence is challenging.
Businesses can resolve this challenge by collaborating with professional data mining firms. The experts can help develop a clear strategy, standardize data formats wherever possible, and leverage ETL Extract, Transform, Load) processes to streamline the integration process. To tackle the complexity and gauge insights for informed decision-making, data mining specialists leverage advanced techniques such as classification, clustering, and association rule mining.
4. Data Interpretability
As data mining algorithms use a combination of mathematical and statistical techniques to uncover trends, patterns, and correlations in the data, they can produce complex models—making them difficult to understand and interpret. Other than this, understanding how the AI/ML model arrived at a particular decision is challenging as it might not be intuitive. This lack of transparency can hinder accountability and make it challenging to assess the fairness and validity of data-driven decisions.
Experienced data mining companies use visualization tools and techniques to represent the data and the models visually, such as heat maps, pie charts, scatter plots, etc. This is because visualization makes it easier to comprehend the trends, patterns, and correlations within the datasets as well as identify the most important variables.
5. Transparency, Bias, and Ethical Concerns
As emphasized earlier, data mining can raise ethical concerns related to how data is collected, used, and shared. For instance, it can be used for purposes that raise ethical concerns, such as discriminatory practices against groups, violating privacy rights, targeted advertising, manipulative nudges, or perpetuating existing biases.
Businesses that outsource data mining should also establish ethical guidelines for such applications, prioritize transparency and user control, as well as avoid using data in ways that could harm or exploit individuals. Experienced professional providers ensure transparency in their data mining processes and algorithms to actively identify and mitigate potential biases as well as avoid making decisions solely based on data-driven predictions without considering human context and ethical implications.
6. Scalability
The volume of data produced had already reached 64.2 zettabytes three years ago, according to Statista. As the data volumes grow, traditional mining approaches might struggle to scale efficiently. The increasing size of data needs more time and computational resources to be processed and analyzed efficiently. Moreover, Machine Learning algorithms must be able to handle streaming data, which must be processed in real-time.
To overcome this challenge efficiently and accommodate the evolving data mining needs of businesses, a professional data mining company uses cloud-based solutions for scalable and elastic computing. You can also leverage distributed computing frameworks such as Apache Hadoop or Apache Spark to meet the scaling needs. By distributing the data and processing it across multiple nodes, these frameworks make it possible to process large datasets quickly and efficiently.
Wrapping Up
Data mining, when conducted responsibly, can be a powerful tool for driving innovation, improving decision-making, and enhancing customer experiences. On that note, challenges such as the ones listed above and others, including user interface, level of abstraction, mining methodology, access to expertise, etc.—should not prevent businesses from uncovering the potential that it holds.
Addressing these dilemmas requires a combination of technical expertise, strategic planning, and ongoing vigilance. By proactively tackling these challenges, organizations can maximize the value derived from their data mining efforts. By investing in the right data mining solutions, they can harness the power of data mining while upholding ethical principles and respecting individual privacy rights by addressing these dilemmas and implementing effective strategies.
Read Also:
- How Much Do Part Time Data Entry Jobs Pay?
- How BI Reporting Is Shaping Modern Business
- Security Tips to Secure your Business’ data